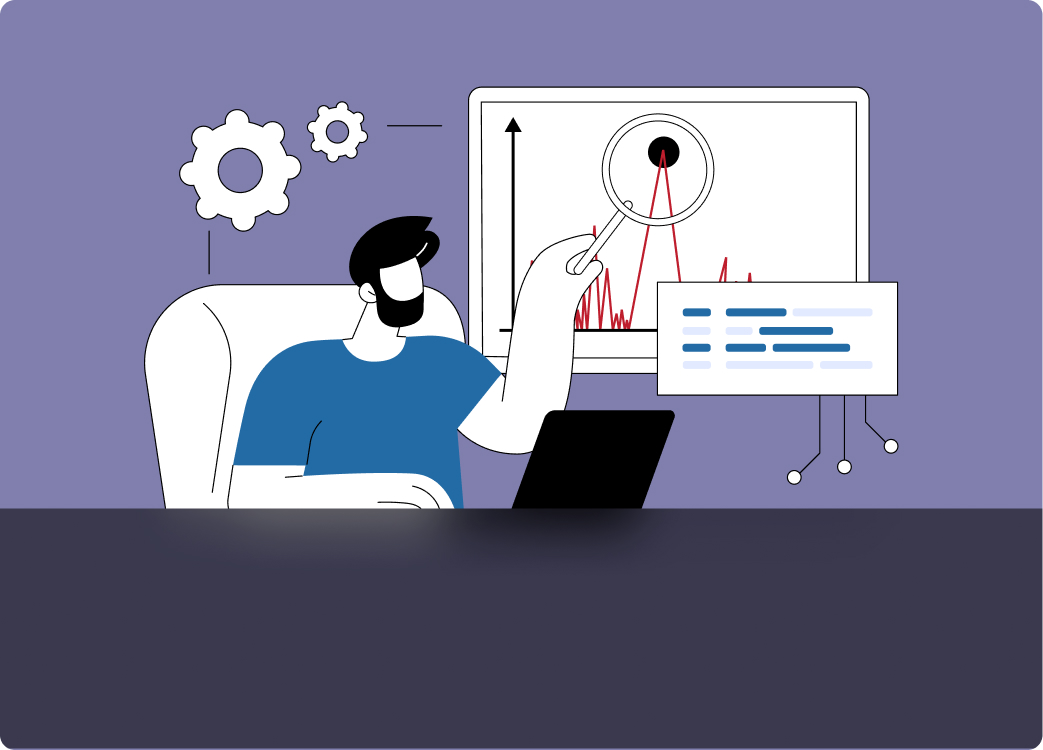
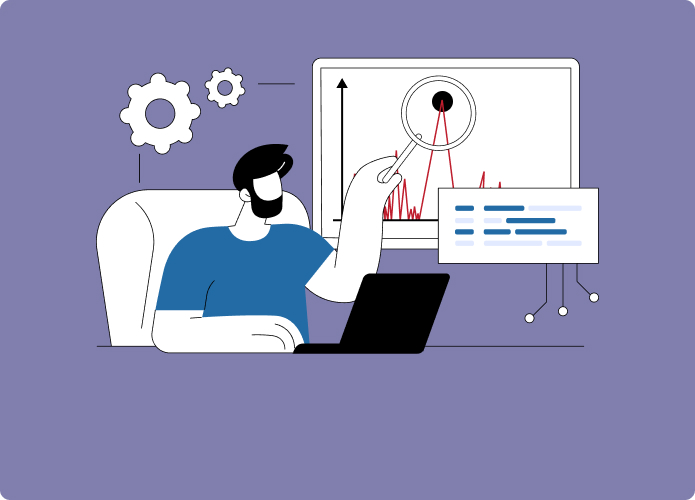
⬤ SaaS
Anomaly Detection vs Traditional Monitoring for SaaS: Key Differences and Benefits
Anomaly Detection vs Traditional Monitoring for SaaS: Key Differences and Benefits
⬤ SaaS
Digital transformation and real-time insights dictate business success today, and effective monitoring is crucial for SaaS companies. Whether it’s ensuring seamless application performance, maintaining system security, or identifying potential bottlenecks, the monitoring approach you adopt can significantly impact your operations.
The debate between traditional monitoring methods and modern anomaly detection systems is no longer about “which is better?” but rather about understanding their unique roles and choosing the right solution for your needs.
What is Traditional Monitoring?
Traditional monitoring has been the backbone of system performance analysis for decades. It operates on predefined rules and thresholds set by administrators, acting as the first line of defense against performance issues.
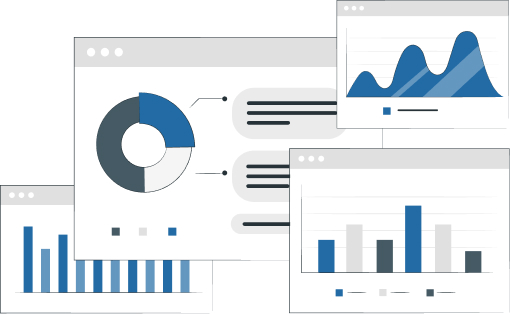
Characteristics of Traditional Monitoring
- Static Thresholds: Administrators set fixed limits for specific metrics such as CPU usage, memory consumption, or response time. For instance, an alert might trigger if CPU usage exceeds the limit.
- Predefined Metrics: Teams select metrics that align with their business goals and infrastructure setup, ensuring that the most critical components are monitored.
- Alerting Mechanism: Notifications are sent when thresholds are breached, allowing teams to investigate and resolve the issue.
Traditional monitoring offers simplicity with easy setup and predictable behavior, making it a cost-effective choice for basic needs and smaller teams.
Limitations of Traditional Monitoring
While traditional monitoring has served businesses well, its limitations become evident in dynamic, data-intensive environments:
Time-Consuming Setup
Configuring and managing thresholds for hundreds or thousands of metrics can be labor-intensive, requiring constant revisions to stay relevant.
Inflexibility
Static thresholds fail to account for natural variations like seasonal traffic spikes or time-of-day fluctuations, often leading to missed anomalies or false positives.
Delayed Response
Manual adjustments to thresholds slow down the response time, leaving systems vulnerable during critical moments.
High False Alert Rate
Without adaptability, static thresholds frequently trigger unnecessary alerts, causing alert fatigue and reducing overall efficiency.
What is Anomaly Detection?
In contrast to traditional monitoring, anomaly detection leverages advanced technologies like AI and ML to provide a smarter, more adaptive approach. Instead of relying on fixed thresholds, anomaly detection identifies unusual patterns in real time.
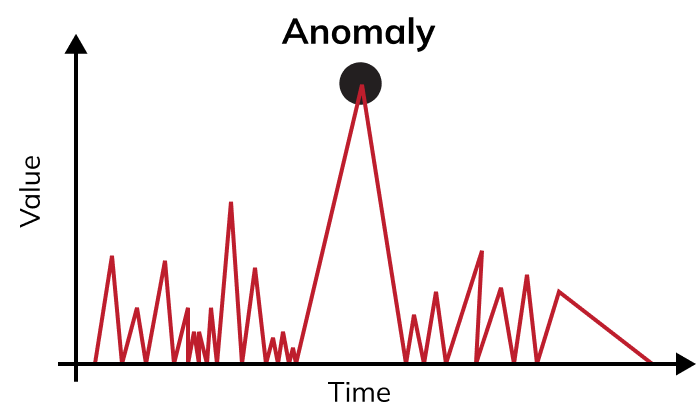
Characteristics of Anomaly Detection
- Static Thresholds: Administrators set fixed limits for specific metrics such as CPU usage, memory consumption, or response time. For instance, an alert might trigger if CPU usage exceeds the limit.
- Predefined Metrics: Teams select metrics that align with their business goals and infrastructure setup, ensuring that the most critical components are monitored.
- Alerting Mechanism: Notifications are sent when thresholds are breached, allowing teams to investigate and resolve the issue.
Anomaly detection ensures early issue detection by identifying deviations traditional monitoring might miss. It reduces alert fatigue by focusing on critical deviations and adapts to dynamic data patterns, enhancing both efficiency and security.
Challenges of Anomaly Detection
- Complexity: Setting up and maintaining an anomaly detection system may require expertise in AI and ML.
- Cost: Advanced tools often come with a higher price tag, which may not be feasible for smaller teams.
- Initial Tuning Period: Anomaly detection systems require time to learn and establish baselines, which can delay immediate effectiveness.
Comparison: Traditional Monitoring vs. Anomaly Detection
To better understand these approaches, let’s break down their differences across key dimensions:
Why SaaS Teams Should Consider Anomaly Detection
The global anomaly detection market is experiencing significant growth, driven by the increasing need for advanced monitoring solutions.
According to a report by Grand View Research, the market was valued at USD 4.33 billion in 2022 and is projected to grow at a compound annual growth rate (CAGR) of 16.5% from 2023 to 2030.
This trend underscores the value of anomaly detection in enhancing security, operational efficiency, and decision-making across industries.
How Anomaly Alerts Can Transform Your Operations
Spot Performance Bottlenecks Before They Become Problems
Ever struggled with sudden slowdowns or unexplained crashes? Anomaly detection helps you identify unusual traffic patterns or performance hiccups early, so you can resolve them before they impact your users.
Stay Ahead of Fraud and Security Risks
Worried about unauthorized access or suspicious activity? With anomaly detection, you’ll catch unusual user behavior that could signal a breach, ensuring your data and your customers’ trust stay intact.
Optimize Infrastructure Without Guesswork
Are you overspending on underutilized resources or scrambling to scale during demand spikes? By tracking resource usage trends, anomaly detection helps you predict future needs and keep your infrastructure lean and efficient.
Understand Your Customers Like Never Before
Have you noticed subtle signs of churn or dissatisfaction slipping through the cracks? Anomaly detection lets you analyze user behavior, pinpoint anomalies, and take proactive steps to retain your customers.
The global anomaly detection market is experiencing significant growth, driven by the increasing need for advanced monitoring solutions.
Conclusion
For SaaS teams, the decision between traditional monitoring and anomaly detection should align with their operational complexity, budget, and goals.
While traditional monitoring may remain relevant in specific scenarios, anomaly detection offers a future-proof solution for modern SaaS operations. By investing in intelligent monitoring systems, SaaS teams can enhance their ability to detect, respond to, and prevent potential issues, ensuring seamless performance and customer satisfaction.
Adopting anomaly detection is not just a technological upgrade; it’s a strategic move toward building a more resilient and proactive operational framework. As the complexity of SaaS environments grows, leveraging advanced monitoring tools will become essential for staying competitive in an ever-evolving digital landscape.
Related Blogs
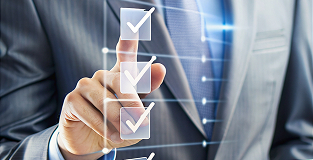
SOC 2 Compliance Checklist
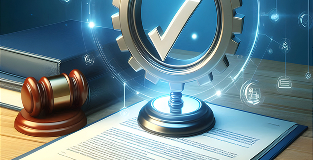
Mastering SOC 2 Compliance: A Complete Guide for SaaS Companies
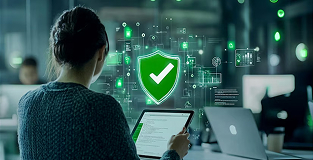